Chapter 11: An Economic Model of General Geological Mapping Applications
Abstract
Geological maps are intermediate public goods that provide information on the surface and near subsurface geology for various applications in different economic sectors. The value of a geological map is inseparable from the expertise used to interpret the map information for a given economic problem. Also, this leads to the critical issue of sufficiency in decision making and the level of investment in an activity. In the case of geological maps, it is rational to only invest up to the needed sufficiency of data collection and interpretation. However, as more detailed mapping is substantially more expensive, the level of sufficiency can be controlled by the available capital. Thus, traditional willingness-to-pay approaches can be problematic in this assessment, and we note that capacity-to-invest may be a more appropriate view. The econometric model indicates that the economic sector and map application are factors for whether the public good form of the geological map is sufficient in most cases. This exercise was not conducted to create a predictive model for value return on geological maps, but rather to understand their position in the economy. As we see from the results, applications in real estate tend to have higher total value than the use in most resource industries, which may differ from assumed conventional views. This study delineates the role and position of geological maps in the U.S. economy and provides insights for future development and investment decisions.
11.1: Introduction
General geological maps (GGM) are a component of the information infrastructure of the U.S. and are viewed as a public good. Here, we evaluate how users of geological maps behave explicitly among map applications in a variety of sectors in the U.S. economy. GGMs contain information on the surface and near subsurface structure, lithology, and other properties, and can be utilized across a spectrum of scales in a wide variety of applications. Geological maps of a given scale can be generalized if an application needs a broader view and operate as a starting point for investigations requiring fine detail.
A wide variety of economic sectors either directly use or utilize the information derived from geological maps, and those uses continue to evolve with the economy. New energy sources, more mobility, and digitization have necessitated changes to the way geological maps are used, distributed, and developed, leading to the need for new development investments. Long-term demands for fossil fuels and related assets are phasing out of the economy, but issues such as the energy transition and its impacts on mineral resource demands and maturing network technology is leading to social changes, such as the acceptance of the remote workplace that have exposed new types of infrastructure asset demands (https://www.mckinsey.com/industries/private-equity-and-principal-investors/our-insights/infrastructure-investing-will-never-be-the-same).
The U.S. and many state agencies provide geological information that has become the standard of accuracy and quality. Specifically, geological maps produced by SGS and the USGS are viewed as highly reliable by 99% and 98%, respectively, of geoscience data users in 2017 (Keane and Mars, 2018). Privately developed and contract maps are viewed as reliable by only 40% of users and are almost exclusively used in the absence of coverage by SGS or USGS products (Keane and Mars, 2018).
However, this study is asking what is the value of a geological map? Given the nature of the place of geological map usage in the economic value chain, we view it as more appropriate to ascertain how to estimate the value of the GGM input in the economy as an intermediate good as its place within the economy. As a result of the survey done for this study, it appears that the market is dynamic and has changed from the historic context in which geological maps were traditionally utilized by the resource industries to a more diverse range of applications.
In this chapter, we investigate the uses of geological map information to reduce costs and uncertainty for economic sectors that are likely to use the map as an intermediate input to a production process. We provide a case for providing geological map information as a public good. Our primary interest is with geological maps that we define as general information at the 1:24,000 scale or broader and are predominately developed using public funds. Then, we develop and estimate the latent demand1 for a range of economic sectors in the U.S. that use scientific information. The latent demand or capacity to invest (CTI) in geological maps is based on how valuable the map can be as an input in the production of a good or service, private or public. In our empirical analysis, the CTI is the dependent variable that we seek to estimate as a function of explanatory variables, while these explanatory variables are economic measures that rely on the informativeness of the science for development activities and for regulatory decisions and actions.
The analysis presented in this chapter represents one of the four approaches taken in the report to analyze the value of GGMs compared to those described in Chapters 6, 7, 8, and 9. It employs the application of an economic model that incorporates the survey conducted for this study. An econometric model is developed to estimate three investment categories of increasing cost to the user of geological mapping as a function of map-use scale, economic sector of application, and the economic dominance of a specific sector in a geographic region.
11.2: Geological Maps as an Intermediate Good
A key consideration in the economic valuation of a geological map is that it behaves as an intermediate good in a value chain. In economic terms, geological maps are used in decision making as an intermediate economic good (Bernknopf et al., 2020). This means that the scientific data contained in the map are one component of a larger value chain that results in the production of a final good or service demanded by society.
A geological map contains scientific data, but the economic value of scientific data is in its interpretation by competent individuals to support decisions based on that distilled new knowledge (Bernknopf et al., 1993). Without the value of skilled interpretation, the value of the map is limited to the physical product materials and production effort. However, with skilled interpretation, which can only be enabled by using the map, the effective economic value becomes far greater, and thus the value of the map is inseparable from the interpretation effort (Bernknopf et al., 1997).
In an example of the value of map information as an intermediate good, Bernknopf et al. (2007) estimated the value of a geological map in the traditional use of mineral exploration. The results of the study demonstrated that the GGM informs the search for Canadian copper deposits. The value of the map is derived as information used by the private sector in the initial search for targets of mineralization. The map contributes to identifying favorable places for detailed industry analysis of the potential monetary return of investment for resource extraction. The study was validated by mineral exploration firms in the industry.
11.3: Materials and Method
As an intermediate good, the value of a geological map is intrinsically linked to the analysis that it facilitates. Figure 11.3.1 is a schematic view of the value dependencies for geological maps. An economic opportunity, regulatory responsibility, or another purpose can generate the need for understanding the physical characteristics of the land surface and resources in the subsurface. The necessity for this understanding is driven by a geological problem that needs to be addressed to facilitate the economic solution.
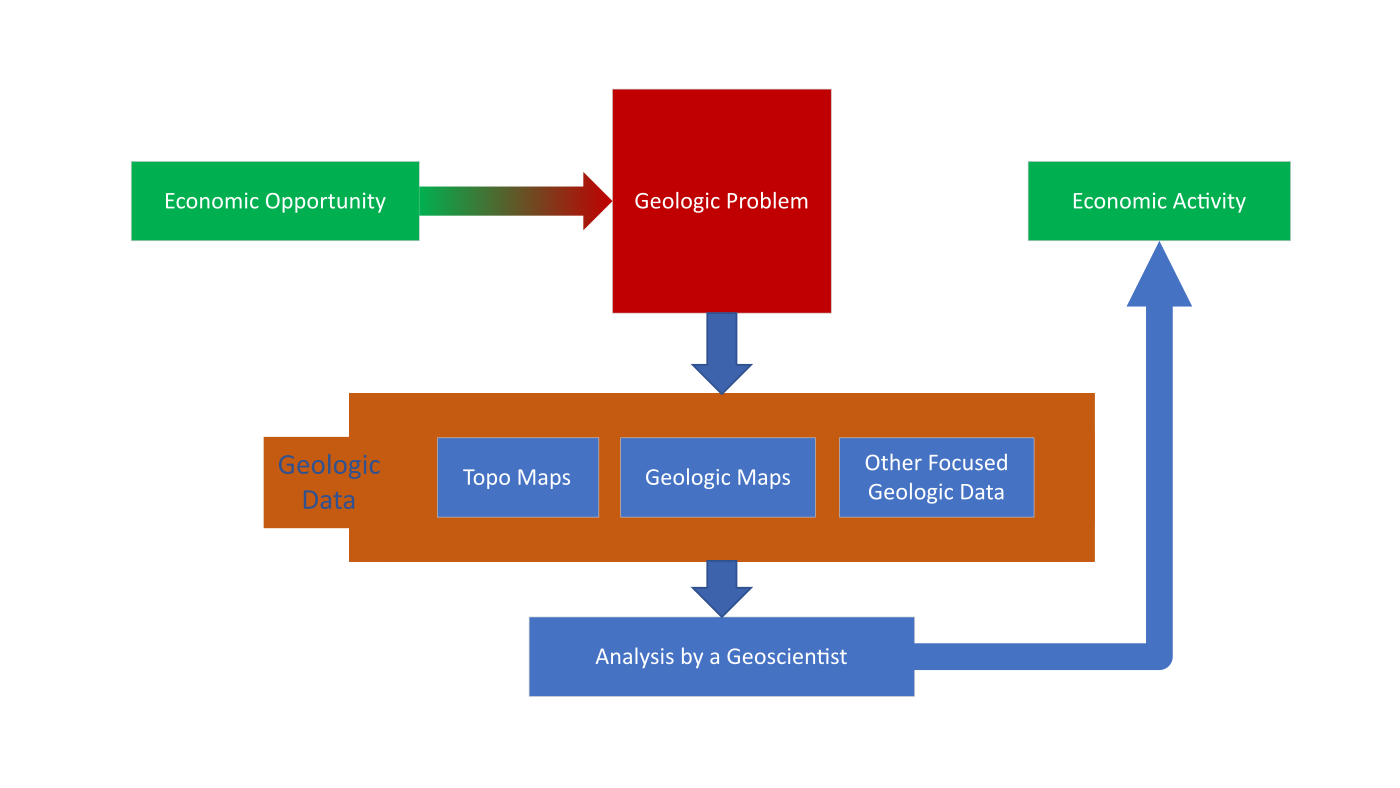
Geological maps are a core asset applied toward the solution. A trained geoscientist will apply the information derived from the geological map and, if needed, other supplemental data can provide an analysis that addresses the geological problem. Hence, the geological information reduces the decision uncertainty involved in the economic activity.
Assuming rational economic behavior, users will be willing to pay for a geological analysis, of which the geological map is a required enabler. In the models that we develop, the value of geological analysis is the intermediate good that is recognized in the decision-making process. That is, the geological map is the combination of field data and scientific interpretation that provides a critical input for the analysis of a societal decision.
To conduct an evaluation of the economic impact of a geological map (Figure 11.3.1), an econometric model is used to identify relationships between variables within the model (Gujarati, 1988). An econometric model is the quantitative analysis of actual economic phenomena based on the concurrent development of theory and observation that are related by appropriate methods of inference (Goldberger, 1964). Econometrics can be further defined as the social science in which the tools of economic theory, mathematics, and statistical inference are applied in the analysis of economic phenomena (Theil, 1971). These definitions suggest that there is a connection between underlying behavioral models and the modern practice of econometrics (Greene, 2012). Applied econometric methods are used to estimate the correlation between user behavior and a suite of user characteristics to analyze the need for policy changes, impacts on markets, testing theories, and for forecasting (Greene, 2012). Here, we apply an econometric model represented as a discrete choice of classes of investment by users of geological map information (Maddala, 1983) against seemingly independent factors that lead to specific decisions.
Geological maps are produced at several scales. Some, such as 1:24,000, are mostly produced by public institutions. Larger scale (i.e., finer scale) maps of small areas and locations are more likely to be produced by entities for specific projects, commonly related to activities in the private sector. We seek to understand the relationship between examples of current map applications and how working at scales generated by public institutions and employed by private entities intersect. Map requirements are likely developed on a project-specific basis, and some combination of both types of maps improve economic efficiency. Through this approach, we attempt to determine whether there are dependencies on sectors and aggregate economic activity that may drive map usage trends, or likewise may represent the greatest utility relative to the perceived value of or the capacity to invest in the geological analysis for decision making on a specific project.
Econometric models are based on statistical regression analysis. The regression model in this chapter is a quantification of the relationship between the dependent variable of user behavior and a set of explanatory factors of user characteristics that can affect the behavior. In other words, in a regression analysis, we try to understand the statistical relationship between a main variable (dependent variable) such as the demand for GGMs and other factors (independent variables) such as map scale and map use in economic sectors. The dependent variable is influenced by chance, whereas the other factors are treated as fixed values that we collect in repeated samples. The econometric model is used to test the hypothesis that there exists a significant statistical relationship between the dependent and independent variables. The intent is to estimate the mean of the dependent variable in terms of the known values of the independent variables (Gujarati, 1988).
The goal of the econometric model is to uncover the causal connections between economic behaviors and the data collected for the independent variables (Greene, 2012). It is also necessary to incorporate stochastic elements. As a result, the observed variation in data can be attributed to two factors:
-
Differences in the variables that are specifically included in the model.
-
The randomness of human behavior along with countless minor influences that are not explicitly considered. (Greene, 2012).
11.4: The Geological Map: Does Map Meet Requirements of a Public Good and an Intermediate Informational Good?
The geological map is defined as (1) a public good and (2) an intermediate good in a value chain in the production of final consumption of economic goods and services. Discussion of the public-good attributes of information involves a distinction between general and specific information. A frequent argument is that general information is a public good, whereas specific information is a private good (Bernknopf et al., 1993). There is the presumption that general information possesses more of the characteristics of a public good, having a lack of exclusion possibilities (anyone can use the information) and a lack of congestion costs (there is no cost of competition in the use of the information).
A public good has two key characteristics: (1) it is impossible, or inefficient, to exclude anyone (nonrival in consumption) from consuming the good once it is produced; the availability to other users is not diminished, and (2) the production of the good is characterized by jointness of supply. Jointness is defined as a physical quality of a good, which allows its consumption by one user to cause no reduction in the amount consumed, at the same time, by others (Musgrave, 1959). Public informational goods are nonrival in consumption. That is, any one individual’s consumption of the map does not reduce the consumption by others.
Geological information can be both general and specific and thus has a different market scope. A GGM contains general information that is constructed from scientific data at a scale and of sufficient informational scope that is valuable in a wide range of land use and land-management decisions such as highway route selection, waste repository siting, and development impacts. Geological maps also are valuable for a long period of time, given the slow rate of decay of its usefulness and that newer products do not supersede the existing information, but rather add new dimensionality. On the other hand, specific information is much more localized and has a lower probability of utility in further application. The collection and use of site-specific geological information for determining the economic feasibility of siting and constructing a multistory office building in an urban center would be of little use in road planning unless the road is to be constructed in the same location as the proposed building. As the information becomes more specific, the number of users becomes smaller.
GGMs also exhibit nonrival consumption. Broader-scale geological mapping enables a wider range of users to use the information over a large area. The approach of broad-scale mapping does not intrinsically lead to legal exclusion of others from making use of the map information unless it is possible to use and enforce developer patents and copyrights. Such rules for exclusion are necessary for the private sector to have the incentive to produce geological map information. Without the imposition of user restrictions, individuals can obtain map information by not paying (a “free ride”) for the information. A private sector producer could not recover the cost of production and would not provide the good. Implementation of an exclusion scheme is difficult in the case of regional geological map information because the range of potential users is large and dispersed; thus there is no way to implement a payment scheme. As information becomes more general, there is a larger group of potential beneficiaries, and there is less likelihood that exclusion is feasible. Therefore, production of geological maps by public agencies emerges due to the excessive cost for private production of broad-scale maps, and the user’s incentive to invest only in fine-scale maps for narrow spatial and application purposes. In addition, public goods become cheaper to provide as more people use them. In the case of GGMs, most of the costs come upfront when collecting and interpreting the data. The costs of giving these maps to more people, like printing or digital distribution, are relatively low.
For example, making a GGM of Loudoun County, Virginia, at the scale of 1:100,000 cost about $1.16 million in 1993 dollars to gather and analyze the data upfront (Bernknopf et al., 1993). But once the map was published in 1992, it cost about $8.44 per copy to print and distribute physical copies. To download from the USGS map archive, there is no cost. So, it does not make sense to limit who can use the map after following its release, because it is efficient or less costly to share it with more people.
The jointness of supply condition of a public good also is fulfilled. That is, a map used by one individual does not reduce the value or utility for other users of the map. The per map production and distribution costs of regional geological map information per single use approaches zero over time as uses accumulate, whereas the actual per unit cost of application to the user is almost entirely in the interpretation enabled by the map in economic and policy applications. The value of a GGM is derived by combining the data and scientific interpretation as an input (intermediate good) in a management or development process to produce a consumption good.
The GGM can be interpreted as a forecast derived from geological data applied in the production of final goods and services (Arrow, 1996). In the valuation process, the geological map is an input factor that influences production and management decisions indirectly rather than a market good that affects consumer utility directly, so scientific information is one component of a larger value chain that can be used to forecast economic outcomes. However, this second distinction of geological map information can limit access to users with fewer resources to participate if there is a limitation, cost or logistical, in access to the necessary expertise to produce the interpretations needed for an application.
The level of expertise needed for a geological map can vary depending on the complexity of the geology and the specific economic application. When it comes to making informed decisions based on scientific interpretation, there is a point at which the information gathered is sufficient to make a practical decision. This sufficiency is related to the scale, detail, or breadth of the analysis (including having adequate data). Similarly, when private entities create geological maps, they tend to focus efforts on their needs.
While the scientific sufficiency of a geological map is crucial, private companies must consider the return on investment (ROI) when deciding how detailed required maps should be and if that entails generating new maps separate from existing public geological maps. A market solution can lead to economic inefficiencies in production if the private capacity to invest is constrained by a decision to produce maps only if ROI ≥ 0. If the return on investment is negative in terms of the cost of science competence required by the problem, it is unlikely that a company will produce a new map. Better capitalized organizations are more likely to be able to invest in generating more comprehensive maps when the ROI is positive, both in terms of data collection and scientific analysis, as their capacity to invest will be higher, but the decision to take action will still be dependent on the expected ROI.
When a firm does invest in producing a geological map, to protect the investment in a competitive market, map creators commonly restrict access to their map through licensing agreements. Importantly, the creation of a private geological map does not prevent other entities, either public or private, from generating their own maps for the same area. This situation creates a challenge in the market, because there is limited incentive to produce comprehensive maps for large areas or for purposes beyond what a specific firm needs that is not adequately addressed by existing public maps. Consequently, it is commonly more efficient for the public sector to provide high-quality geological information to a wide range of users in a fair and equitable manner.
11.5: Economic Models for Valuing Geological Maps
To assess the value of an intermediate public good for individuals, economists rely on two main approaches: revealed preference (RP) and stated preference (SP) models, both aimed at assigning a monetary value to the good. RP methods involve studying consumer behavior to estimate the marginal value of the good. This approach identifies how people act in real-life situations to determine the worth of the intermediate public good (Freeman, 2003). On the other hand, SP techniques also aim to gauge changes in well-being and estimate the prices individuals are willing to pay for goods. However, SP differs from RP analyses, because it does not rely on directly observed market behaviors or monetary transactions to determine value but, instead, it gathers information through surveys and hypothetical scenarios to understand preferences and willingness to pay (WTP).
RP models use Benefit-Cost Analysis (BCA) that systematically categorizes the benefits and costs to estimate the net benefits, and this includes the proposed geological map information compared to a baseline information case. We assume there is existing geological map information available that is older that would represent an outdated scientific interpretation. To do this, RP models gather scientific and technical data related to the geography and geology in question. These data are crucial for understanding how the geological map influences decision-making. Monetary values are assigned to these benefits and costs through specific case studies, forming the foundation for what is known as the “Value of Information.”
SP models, on the other hand, involve surveys of current and potential users of geological map data. These surveys aim to gauge the WTP by users for access to improved information. Questions in these surveys may revolve around the potential savings users expect from reduced time, labor, and informed decision making. These surveys can take various forms, such as face-to-face interviews, telephone interviews, online surveys, mail surveys, or some combination. SP techniques depend on asking individuals hypothetical questions to create a hypothetical market (Pearce et al., 2002).
In SP models, the economic value is determined by the responses to these survey questions, which provide an estimate of how much individuals are willing to pay to access an intermediate public good (like geological map information) that directly impacts their well-being. This WTP is a measure of how much someone is willing to spend to improve the state of a particular good compared to leaving it as is, essentially capturing the value they place on this improvement (Freeman, 2003).
SP techniques can be controversial for economic valuation, because survey respondents put themselves into hypothetical situations and potentially react differently than in real transactions (Freeman, 2003). In these types of circumstances, there is evidence that consumers tend to overestimate the value of the good or service being evaluated (Freeman, 2003). WTP surveys are effective but costly. However, if a WTP survey is undertaken, considerable attention is needed to eliminate bias from the survey to preserve the credibility of the results. Several potential sources of bias exist in these kinds of studies. The surveys can ask users to estimate the benefits they would experience from updated GGMs that do not actually exist. Answers to such hypothetical questions may present a problem known as hypothetical bias. Furthermore, since map users are able to benefit from new mapping, there may be a strategic bias in which individuals may report a higher level of expected benefits from new development projects to influence any decisions regarding the project (Bernknopf et al., 2020).
The intended outcome of this study is to evaluate the WTP for a geological map by the map-user market as a measure of its value to society and the economy. The core dataset is from a survey based on a SP model, and this econometric analysis is limited to the results of the survey. Survey responses included a variety of variables, including scale, economic sector, location, and data on value ranges. Use of the WTP by the respondents posed challenges for developing an economic model to identify the factors impacting decisions on the level of investing in acquiring map information. Consistent definitions of spatial extent and cost basis required identifying an effective WTP proxy metric, which for this model, broke between the medium and small-scale maps generally produced by public entities and the detailed scale that are more likely produced by private mapping efforts. The survey responses are not independently observed actual market transactions related to the acquisition of geological maps, but rather cost statements or value assessments for a mix of existing, potential, and declined activities. A range of responses were received, which may reflect some variation by respondents in the interpretation of the questions and descriptions of project scope.
The survey responses did shed light on behavioral thinking about the role of geological maps in solving economic issues. Some respondents reported on direct nominal acquisition costs of geological maps for use in their projects. Others reported on values representing the totality of the intermediate good, including the geological analysis leveraging the maps. With the discussion of use of multiple map scales, varied spatial extents, and similar factors, for the purposes of the economic analysis, we recognize the sufficiency issue in geological analysis, and thus the response may better reflect a capacity to invest than a traditional willingness to pay.
In any problem-solving process, there is a threshold of information sufficiency to make a confident decision. This information, in the case of geological maps, is an integrated combination of data, analysis, and scale. There is likely a continuous probability curve representing certainty of a correct decision based on data and analytic intensity applied to the solution for geological mapping, scale, and detail of the map.
Given that many geological-economic problems represent costs such as direct application of the information or liabilities that need to be offset (such as meeting environmental regulations or engineering standards), there is a disincentive to overinvest in the analysis. In scenarios of sufficient capital to address a cost-centric problem, the reported value will represent a willingness to invest. Likewise, if the capital available is limited, as would be expected in most real-world situations, the actual value reported on data and analytic input reflects the capacity to invest from which point there will be a defined certainty of a correct solution decision.
11.6: An Econometric Model of Map Applications
This project assessed the value of geological mapping through multiple methodologies. The approach in this chapter, utilizing an econometric model, is focused on understanding the importance of geological maps as a significant input factor in a production process in an economy and what might be influencing it in that role. The model is meant to reveal general structural trends and is not a precise predictive tool or one that provides nuanced estimates of the exact magnitude of influence of the independent variables.
Our interest is estimation and the analysis of a specific model to ascertain the influence of factors for deciding the mapping investment for a given problem. As mentioned previously, the responses reflected the actual use of geological maps, which does not necessarily reflect a traditional willingness to pay, but likely limitations on a capacity to invest that yields a defined certainty level. Within narratives in the responses, we see examples of descriptions of work abandoned because of inability to invest enough to obtain informational sufficiency. As such, the economic value of the map information informs value-added production costs of a final consumptive good or government policy choice.
In this analysis, we assume that geological map users are competent and apply appropriate scales, based on the nature of the problem to solve and the ability to have funds to acquire the data and interpret the data to produce the needed geological maps. Furthermore, we assume that public institutions produce public good maps and likewise preferentially use them.
11.7: Transformation of Data for Econometric Analysis
The survey responses provided a wide set of variables for consideration in performing an econometric analysis. One characteristic of many of the survey responses was individual fields of a given response could contain multiple discrete answers, such as scales of maps used. Additionally, individual respondents were not always clear in their answers whether the responses reflected the activities of a specific project or that of the whole of the responding entity, an observation supported by reviewing associated narrative response fields. To facilitate analysis, responses were “unrolled” such that for each response, a new data row was created representing each possible perturbation reflected in the multivalued response fields. Though this approach negatively impacts the statistical strength of the analysis, it also reduces the relational power between variable values given the imprecision of the original responses. For the sake of analysis, each of these unrolled data rows was recognized as a distinct observation in the dataset.
For the economic analysis, we filtered the unrolled dataset to only consider complete responses in which no fields of data were missing. This filter yields an analytic dataset of 2,937 responses. Each response had data covering a range of factors, including location (by state), map scale used, dollar value brackets for the activity, economic sector of the activity including state and local government, federal government, real estate industry, energy and mining industries, education, and professional services.
11.8: Model Variables
To assess the factors that influence the demand for geological maps, we have utilized the survey WTP responses as discussed in Chapters 6 and 10 to undertake an econometric analysis. Demand for geological information is represented as the capacity to invest (CTI) in the production of geological map information from public, private, or both sectors. However, a latent demand exists in the form of a consumer’s CTI for map production that is based on the SP responses explained in Chapters 6 and 7.
Our analysis also assumes that those who participated in the survey are the best sample of individuals to assess the CTI for geological maps. The survey is not comprehensive in coverage of all users of geological maps, and we have assumed users not represented in the survey sample have preferences aligned with the respondents of the survey as otherwise may be limited, as explained in Chapters 2 and 6.
As the primary point of the overall study is the estimation of the economic value of geological maps by consumers, we considered an econometric analysis that would represent an approximation of the consumer decision process in paying for geological maps — namely whether they are using preexisting maps or generating new maps, or some potential combination of both.
We conducted an econometric analysis to establish an economic variable of mapping choice behavior. Survey respondents indicated a WTP for each map, with response options binned as:
- < $1,000
- $1,000–$5,000
- $5,000–$10,000
- $10,000–$25,000
- $25,000–$50,000
- $50,000–$100,000
- $100,000–$200,000
- $200,000–$300,000
- $300,000–$400,000
- $400,000 and higher
We analyzed the distribution of responses and found distinct populations in the low end ($5,000 or less) and the high end ($25,000 or larger). We have assumed that these WTP levels are indicative of behavior toward the mapping needs of the respondents.
The dependent variable in the model implies that a latent demand is associated with the CTI for an input cost of geological map information to produce a final good. The CTI in the econometric model is represented by three options derived by the indicated WTP from the survey as clustered into three probable cost-behavioral brackets:
Off-the-Shelf: For responses with a survey response to WTP of $5,000 or less, we classified the response as choosing an off-the-shelf map, as this expense might cover nominal acquisition costs of public maps or commercially available data through a vendor but is distinctly insufficient for original mapping of any material extent. About 40% of the responses were in this value range of $5,000 or less.
Custom Maps: These data are for responses with valuations that are $25,000 or larger, which is a cost scope that can represent material professional investment of time and resources, likely more than 25% of a professional FTE. We expect these responses involve either active augmentation of existing geological maps and/or the development of new maps at standard or site-specific scales. About 20% of responses were in this value range of $25,000 or more.
Transitional Mapping: These observations are projects that reported values between $5000 and $25,000, representing an intensive investment in the geological data component, but not likely to the level of extensive custom mapping. We hypothesize that many of these responses are of either large spatial extent with multiple analysts and/or required the selection of additional mapping detail to address specific issues to ensure scientific sufficiency for the problem.
The independent variables utilized in this econometric model included reported classification of the use of a map scale, the economic sector reported for the application, and the per capita GDP attributed to each of the reported sectors.
Map Use Scale: Each response indicated the map scales used in their response — 1:5000, 10,000, 24,000, 100,000, and 500,000. Each map scale represents an application space, and the map scale was coded as local scale (1:5000 and 1:10,000), general utility scale (1:24,000 or smaller), and cross scale if they indicated use of maps within both bins. As few public maps are generated at greater than 1:24,000 scale, local scale and cross scale responses are likely indicative of at least some private mapping.
Economic Sector: Responses indicated the economic sectors of application: state/local government, federal government, real estate, education, energy, mining, transportation, and professional services.
Per Capita Sectoral GDP: An additional economic factor used in the econometric model captures a measure of the economic dominance of a specific sector in a geographic region. We characterize this explanatory variable as a per capita sectoral GDP, i.e., the associated GDP component of the economic sector normalized by the population of the region. As described in Chapter 8, the regional variation in the economic responses to the survey were limited, inferring that geological map values have little specific regional dependency overall, but that does not indicate if the proportional economic activity, which varies by region, might be a factor. For example, real estate is not as large an economic driver in Nevada compared to Maryland because of differences in population density, but mining is a bigger part of Nevada’s economic activity than in Maryland because of the availability of extractive resources.
11.9: Econometric Analysis
The econometric analysis is initiated with a statement of a theoretical proposition. In the developed model, our hypothesis is: Geological map information is economically valuable to public and private economic decisions.
Geological map information is derived from publicly observable phenomena, as it is intrinsically a public good since the fundamentals of a map are non-rivalrous and non-exclusionary. Geological map information should be provided as a public good because of the many uses of general information and the inefficiency to exclude anyone (nonrival in consumption) from consuming the product as a market good.
The concept of multiple regression constitutes the underlying platform for our multinomial choice model. A multinomial choice model assumes that individual behavior is to choose among more than two choices and seeks to make the choice that provides the greatest utility. As defined above, the application is the decision-maker’s choice among three investment alternatives of increasing levels of user investment for geological map information. These choices define a ranking of the CTI for geological information. The three classes of investment in producing reliable geological map information include: (1) off-the-shelf maps provided mostly by SGS and USGS or public data vendors (least costly); (2) transitional map projects that combine public sector maps and user staff/contractors (some cost to user); and (3) custom mapping that uses internal staff and/or contractors (costliest).
A multinomial logistic model is employed to estimate the probability of user type to invest in geological information by imposing the logistic distribution on the qualitative choice (Greene, 2012). Estimation of the parameters of the chosen model is the chance that a user is in one of the three categories of CTI for a geological map, which reflects the amount invested in a map application as an input in the form of an informational intermediate good. The model uses the CTI as the dependent variable and uses the survey questions on map scale use (MSU), economic sector of application (ES), and our calculated per capita GDP of the application sector for 2019, the year of the survey (GDP) as a measure of potential aggregate available capital in a sector.
The model at national scale is:
Capacity To Invest (CTI) = Map Scale Use (MSU) + Economic Sector(ES) + Per Capita Sectoral GDP (GDP) + e (1)
where e is a statistical error term.
Table 11.9.1 presents the estimated parameters and model verification through statistical inference. These estimates provide empirical evidence that aligns with the economic theory, reinforcing the idea that a geological map qualifies as a public good (Gujarati, 1988).
To arrive at these estimates, we employed an ordered logistic regression model using the unrolled survey responses comprising 58,191 observations from the year 2019. The verification of our model hinges on assessing whether it aligns with the expectations set by the theory under examination. We subject the results to statistical inference tests to determine their credibility, significance, and to rule out the possibility that they are merely the result of random data sampling.
Results are shown for variable coefficients, standard errors, and inference statistics in Table 11.9.1. The Z-statistic tests if the effect of the variable (coefficient) has no effect (Z = 0). A larger absolute Z value is indicative of an effect. The sign of the coefficients indicates whether that variable increases the probability of making a specific decision on CTI (positive coefficient) or increases uncertainty (negative coefficient). The P > |z| statistic is a measure of the probability of the predictive influence of the z value.
Table 11.9.1. National Scale Ordered Discrete Choice Model of Three Categories of the Capacity to Invest in Geological Map Information
Coefficient | Standard Error | z–value | P > |z| | Confidence Interval [0.025–0.975] | |
Local Scale (≤10"K" ) | −0.3096 | 0.021 | −14.534 | 0.0 | −0.351–0.268 |
Regional Scale (24K) | 0.4372 | 0.011 | 40.605 | 0.0 | 0.416–0.458 |
State Local Govt | 0.0985 | 0.074 | 1.324 | 0.185 | −0.047–0.244 |
Federal Govt | −0.0212 | 0.023 | −0.915 | 0.36 | −0.067–0.024 |
Real Estate | −0.041 | 0.117 | −0.351 | 0.725 | -0.27–0.188 |
Education | −0.0581 | 0.04 | −1.465 | 0.143 | −0.136–0.02 |
Energy | 0.0833 | 0.039 | 2.153 | 0.031 | 0.007–0.159 |
Mining | −0.0324 | 0.041 | −0.796 | 0.426 | −0.112–0.047 |
Professional Services | −0.0959 | 0.023 | −4.238 | 0.0 | −0.14–0.052 |
geopercapita | −7.37E-05 | 2.01E-05 | −3.66 | 0.0 | 0.0–3.42E-05 |
- Log-Likelihood: −60238
- AIC2 : 1.21E+05
- BIC: 1.21E+05
- Number of Observations: 58,191
- Degrees of freedom Residuals: 58,179
- Degrees of Model: 12
The model in equation 1 establishes national level relationships between map-use characteristics and the choice of mapping used: off-the-shelf, transitional, or custom. Inspection of Table 11.9.1 revealed that map scale use for local or district only criteria had extreme predictive power relative to the multiscale use default in the categorial framing of the independent variable. As the CTI bins were defined on expectations of using available maps (off-the-shelf) to custom maps that are more likely local in scale, this relationship appears rational. Site-specific information from local scale (10,000), which is more likely to have a specific application, has a negative and significant impact on CTI*.* This phenomenon may be due to the limitation of land use to an individual project. On the other hand, district scale geological maps (1:24,000) have the opposite effect. By design, a broader geological map contains more general information and is of greater use to more users in specific economic sectors. This variable is positive and significant in predicting the specific CTI in maps in both private (e.g., locating investment focus by comparing regional location options) and public (e.g., regulation of land use and land cover for economic development) sector applications.
Specific economic sectors had varied predictive value of the geological map input factor in predicting the three CTI mapping options. For example, professional services associated with the application of extant geological maps is negative and significant as expected, predictive z value = 0.0 as seen in Table 11.9.1. Given the important position of the professional services sector in the application of geology, this finding is not unexpected. Currently, 41% of all geoscientists in the U.S. are employed in the professional services sector, and their work is focused on applying appropriate professional knowledge to the problem. Based on this professional knowledge, the geoscience includes an expected rationale for right sizing of the geological mapping needs, but these needs are also unlikely to be capitalized to produce custom maps of any major scale.
The energy sector exhibited a significant and positive relationship with CTI that would be due to the geographic constraint of resources and the infrastructure needed for exploration, production, and distribution, yielding a p-value of 0.031, and thus significantly different at the 95% confidence level. Additionally, the energy sector relies more heavily on subsurface geophysical data, coupled with depressed domestic onshore exploration demand during the time of the survey. This is not true for the mining sector (i.e., minerals industry). Not only is the minerals industry in active exploration activities, but the inherent nature of its work is also more site specific except for regional evaluation that can focus on identifying exploration targets, which yields a non-significant probability of 0.426.
Governments are, for the most part, users of public good maps. Federal users rely on SGS and USGS maps and are unlikely to invest their own resources, resulting in a probability of 0.36. While in the case of state governments and local governments, institutions also would use district maps from federal and state map producers, with a non-significant probability of 0.185. Real estate users are unlikely to produce their own maps due to the specific nature of investments with non-significant probability of 0.725. Educational institutions are likely to use off-the-shelf maps for instruction and site-specific information for specific research projects with low, but not fully significant probability of 0.143.
The final independent variable, Per Capita Sectoral GDP (GDP) is negative and highly significant, with a probability of 0.0. This relationship is due to the types of industries and land uses that dominate the national landscape. To reiterate, the model represents a national level set of results, and regional responses vary.
The econometric model indicates that the application scale and wealth of the application sector are drivers for a capacity to invest. Problems that are rationally recognized as needing custom mapping make a better argument for increased capital investment. Likewise, sectors with great economic power are also likely to face less friction on investment to meet the sufficiency needs, especially in problems requiring finer scale mapping information.
11.10: Results: Use of Geological Maps is Measurably Rational
With the driving mechanism of the economic activity related to the application sectors, we extended our analysis to evaluate how the economic value of each sector can be expressed by the various levels of investment by that sector in geological mapping. This is propelled partly by existing biases within the geological community related to the importance of the resource sectors in the overall demand and economic return for geological maps.
Using the GDP component of each sector, as provided by the US Bureau of Economic Analysis for 2019, we identified the sectoral contribution to per capita GDP. Then, analyzing the actual survey response rates by economic sector and whether the project used off-the-shelf, transitional, or custom mapping, the allocation of each mapping type was calculated for the sectoral GDP. We assume that the proportion of map types (off-the-shelf, transitional, and custom) would apply to the economic sector nationally, regardless of the scale of activity within that sector in 2019.
Table 11.10.1 shows the results of this analysis and especially the value recognized that is clearly the public good in the off-the-shelf category, representing 54.7% of the GDP-weighted value of all geological mapping, and for projects that likely included all or some publicly produced geological maps, representing 75.6% of the economic value, or $19,243 per person for 2019.
Table 11.10.1. Sector Per-Capita Allocated by Rate of Map-Scale Use Profile
Sector | Off the Shelf | Transitional | Custom |
Mining | $131.52 | $133.47 | $94.01 |
Energy | $256.63 | $190.34 | $134.03 |
Real Estate | $4,781.26 | $1,218.54 | $2,196.20 |
Construction | $1,193.58 | $674.08 | $1,006.34 |
Professional | $1,432.67 | $1,033.70 | $687.64 |
Transportation | $754.53 | $354.74 | $395.72 |
Education | $569.16 | $257.33 | $47.50 |
State/Local | $3,485.42 | $1,216.62 | $1,056.96 |
Federal | $1,316.90 | $242.95 | $607.15 |
A further point of discussion is the distinct regional applications of geological maps. Using this same approach, we analyzed the data to look for regional dependency on the scale-value proposition. We examined the ratio of use of local (and likely custom) maps to regional (and likely off-the-shelf) in the responses for each region and compared those to the ratio of use of local (and likely custom) maps to the sum of known regional and cross-scale maps, which will include the publicly produced maps.
This second ratio focuses on the level of use of solely custom mapping to the utilization of the public good. Table 11.10.2 shows the results of this analysis. In general, public good maps are used 8-to-12 times more frequently than custom maps in most regions. However, there are two notable regions where this trend deviates. The South Central, which is dominated by energy sector responses, has distinctly higher rates of custom map use, likely reflecting the nature of the applications, namely focused on engineering and environmental site assessments for energy infrastructure such as wells and pipeline footings. Similarly, the use of custom maps in the Northeast versus strictly off-the-shelf maps is inverted, indicating a much higher use of custom mapping. A likely driver of this phenomenon is the large demand for geological maps in real estate, and when coupled with the high property values in the Northeast, capital for more local geological mapping may be available, especially with numerous building codes to address issues such as radon or the redevelopment of industrial brownfields that necessitate greater geological detail for assessment.
Table 11.10.2. Regional Applications of Geological Maps
Region | Local:Regional | Custom Usage |
Great Lakes/Great Plains | 0.14 | 0.04 |
Intermountain West | 0.22 | 0.07 |
Northeast | 1.43 | 0.27 |
Pacific Rim | 0.13 | 0.04 |
South Central | 0.64 | 0.20 |
Southeast | 0.21 | 0.06 |
Table 11.10.3 presents data on how geoscience-influenced sectors intersect regionally, represented as a percentage of the regional GDP for 2019. Using the identified sectors in the econometric model as reported sectors of application of geological maps, the regional contribution to GDP from the U.S. Bureau of Economic Analysis of those sectors was evaluated against the total regional GDP that is calculated as a first-order estimate of potential economic influence of geoscience by region in the U.S. For instance, in the Great Lakes region, geological maps are used in sectors impacting just under 34% of the GDP, whereas in the Mountain West, it affects over 43%. While the specific applications of geoscience can differ widely, many sectors within these regional economies derive significant benefits from geological maps.
Table 11.10.3. 2019 Regional Percentages of GDP Impacted by Geoscience.
Calculated from U.S. Bureau of Economic Analysis data
Region | % of GDP Geoscience Impacted |
Great Lakes/Great Plains | 33.7% |
Intermountain West | 43.2% |
Northeast | 37.8% |
Pacific Rim | 37.3% |
South Central | 40.6% |
Southeast | 38.4% |
11.11: Summary
Geological maps are an integrated product of scientific data, analysis, and interpretation. The information value can be considered non-separable among these components that are used to create the value of a geological map. The data and the science provide an intermediate public good. SGS and the USGS provide the intermediate public good to public agencies and private economic sectors.
Based on the characteristics of an informational intermediate good, geological maps have their greatest economic value if produced as a public responsibility to provide accurate and informative information to maximize the number of uses and users as possible. Furthermore, geological maps as a public good is not an endpoint but rather an intermediate good of production that supports economic sectors and is useful in regulatory and land status decisions.
Geological map sufficiency is based on the adequacy of the map and the scientific competence of the map maker to address the economic decision problem at hand in the application. As reported, the market for maps indicates that geological maps produced by the SGS and USGS provide sufficient detail, reliability, and consistency to make actionable and supportable decisions. While scientific sufficiency of geological maps is critical, a private capacity to invest to produce a map comparable to the public good map has a limiting threshold that is based on the required return on investment for a particular firm.
A range of logistic multinomial regressions were estimated and tested to establish the capacity to pay for a geological map. The statistical analysis of the CTI affirmed national consistency using a full, but sparse dataset. Aggregate behaviors of respondents were generally very consistent across all regions of the U.S. We found regional differences, such as the demand for finer scale mapping in the Northeast U.S. for issues such as radon requirements in the real estate sector. Additionally, we found that lower sector per-capita values are more likely to use the less costly off-the-shelf maps.
The econometric model identified behavioral relationships in the stated preference dataset. However, there are limitations to the analysis. In conducting a range of model regressions, various model runs generated identity matrices. There were also models containing internal perfect correlation between independent variables, which was problematic. Also, there were models that exhibited instability from data sparseness. Additional model runs are available upon request.
We have developed a macroeconomic model that rationalizes the production of sufficient geoscience as a public good by both SGS and the USGS. The capacity to invest demonstrates how the size of economic entities influences the availability of geological information and equal access to a fundamental part of the U.S. data infrastructure. Finally, further research on the value of geoscience information at the Congressional district level would provide significant support for the public sector supply of geological map information.
11.12: References
Arrow, K., 1996, The Economics of Information: An Exposition: Empirica, v.23, p. 119–128.
Bernknopf, R., Brookshire, D., Macauley, M., Jakeman, G., Kuwayama, Y., Miller, H., Richardson, L., and Smart, A., 2020, Societal Benefits—Methods and Examples for Estimating the Value of Remote Sensing Information: Manual of Remote Sensing, 4th Edition: American Society for Photogrammetry and Remote Sensing, p. 869–910.
Bernknopf, R., Wein, A., Lucas, S., and St-Onge, M., 2007, Analysis of Improved Government Geological Map Information to Mineral Exploration: Incorporating Efficiency, Effectiveness and Risk Considerations: USGS Professional Paper 1721 and Geological Survey of Canada Bulletin 593.
Bernknopf, R., Brookshire, D., McKee, M., and Soller, D., 1997, Estimating the Social Value of Geologic Map Information: A Regulatory Application: Journal of Environmental Economics and Management, v. 32, p. 204–218.
Bernknopf, R., Brookshire, D., Soller, D., McKee, M., Sutter, J., Matti, J., and Campbell, R., 1993, Societal Value of Geologic Maps: U.S. Geological Survey Circular 1111.
Freeman, A., 2003, The measurement of environmental and resource values: Theory and methods (2nd ed): Resources for the Future, 478 p.
Greene, W, 2012, Econometric Analysis, 7th edition: Essex, U.K. Pearson Education Limited, 1232 p.
Gujarati, D, 1988, Basic Econometrics, 2nd edition: New York, McGraw - Hill Publishing Company, 705 p.
Keane, C., and Mars, M., 2018, The Extent of Geologic Map Data’s Impact on the U.S. Economy: Geological Society of America Abstracts with Programs., vol. 50, no. 6, ISSN 0016–7592; DOI: 10.1130/abs/2018AM-316758.
Maddala, G, 1983, Limited — dependent and qualitative variables in econometrics: United Kingdom, Cambridge University Press, 414 p.
Musgrave, R, 1959, The theory of public finance: New York, Macmillan.
Pearce, D., et al., 2002, Economic valuation with stated preference techniques: Summary guide: London, Department for Transport, Local Government and the Regions, 87 p.
1 A demand which the consumer is unable to satisfy, usually for lack of purchasing power (https://www.kamcity.com/kamwords/demand-latent/).
Table of Contents
- Abstract
- Acknowledgements
- Executive Summary
- Chapter 1: Introduction
- Chapter 2: Study Objectives and Methodology
- Chapter 3: Stakeholder Assessment of Map Producing Agencies
- Chapter 4: Cost for Geological Mapping
- Chapter 5: Geological Mapping Program Activities — Critical Components
- Chapter 6: Benefits of Geological Mapping: Quantitative Assessment of Responses to Stakeholder Questionnaire
- Chapter 7: Geological Map Demand and Economic Estimates of Costs and Benefits
- Chapter 8: Regional Variations in Costs and Benefits of Geological Mapping
- Chapter 9: Quantitative Value Assessment from Independent EPA Data
- Chapter 10: Qualitative Assessment of Value of Geological Maps by Stakeholders
- Chapter 11: An Economic Model of General Geological Mapping Applications
- Chapter 12: Stakeholder Input about Future Geological Mapping
- Chapter 13: Lessons Learned and Suggestions for Future Analyses
- Chapter 14: Summary and Conclusions
- Appendix 1: Cost Sheet Template
- Appendix 2: Questionnaire to Stakeholders
- Appendix 3: Example Solication Letter Requesting Stakeholders to Participate in National Cost-Benefit Assessment
- Appendix 4: Summary Statistics, Outliers, and Confidence Intervals
- Appendix 5: Annual State Geological Survey Map Views
- Appendix 6: Regional Cost-Benefit Analysis Datasets
- Appendix 6a: Questionnaire Data Schema
- Appendix 6b: State Geological Survey Reporting Schema
- Appendix 7: Chapter 8 Supplemental Figures and Tables